
A large variety of computational methods have been developed for this purpose, exploiting various data sources ranging from MEDLINE abstracts, functional gene annotation, protein–protein interactions and high-throughput gene expression data to intrinsic gene or protein properties (such as coding sequence length, number of introns, conservation and so forth). Finally, we present novel disease-gene predictions for eight loci associated with different syndromes of unknown molecular basis that are characterized by mental retardation.Ĭontact: or information: Supplementary data are available at Bioinformatics online.Īlthough experimental methodologies for the identification of disease-causing mutations have significantly improved-in particular by the recently introduced next-generation sequencing techniques-computational approaches to an in silico evaluation of candidate genes remain an important aid for the identification of genes involved in human hereditary disorders ( Kann, 2010 Piro et al., 2011 Piro and Di Cunto, 2012).

Results: We improved our previous work 2-fold: (i) we demonstrate that condition-independent transcription factor binding affinities of the candidate genes’ promoters are relevant for disease-gene prediction and can be integrated with our previous approach to significantly enhance its predictive power and (ii) we define a novel similarity measure-termed Relative Intensity Overlap-for both 3D gene expression patterns and binding affinity profiles that better exploits their disease-relevant information content. 3D, gene expression data from the mouse brain can be successfully used to prioritize candidate genes for human Mendelian disorders of the central nervous system. We have shown recently that spatially mapped, i.e. Several excellent methods for disease-gene prediction have been developed in the past 2 decades, exploiting widely differing data sources to infer disease-relevant functional relationships between candidate genes and disorders.
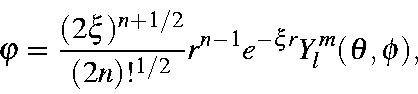
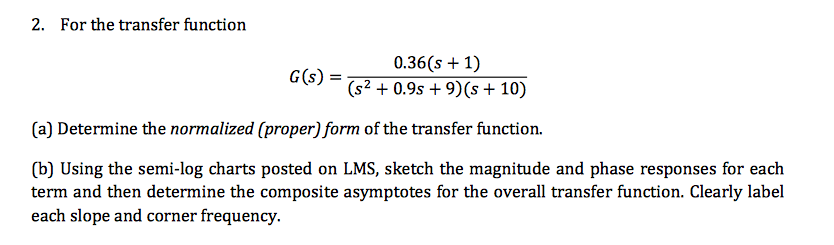
Motivation: The computational evaluation of candidate genes for hereditary disorders is a non-trivial task.
